Outline of research
The fragment molecular orbital (FMO) method, originated in Japan, is an ab initio quantum method that gives detailed information of interaction between a compound and the target protein based on electronic state calculations.
With its high reliability and effectiveness, FMO is expected to advance logical drug design much further than conventional methods. Since the proposal of FMO by Kitaura et al. in 1999, a vast of research projects have been performed on both theory and application of this method. These fruits of research can be turned into practical drug design by collaboration of academia and industry. Thus launched FMODD in November 2014, aiming to further accelerate the use of the FMO method in in silico drug design while taking advantage of “High Performance Computing Infrastructure” (HPCI). In FMODD, FMO researchers work together with drug designers from private companies to develop and spread practical application of FMO.
In FMODD, we share the following know-hows of FMO calculation and analysis, as well as the data obtained with FMO:
1. Prediction of binding characteristics between proteins and ligand molecules for ligand screening,
2. Combination of FMO with other in silico methods such as docking, molecular dynamics, and bioinformatics,
3. Interpretation of experimentally obtained molecular structures, as well as structure refinement, using FMO,
4. Construction of the FMO database (FMODB) to archive electronic structures of biological molecules,
5. Prediction of molecular properties from large-scale FMO data by machine learning and AI, and
6. Development of Molecular Mechanical (MM) force field fitted to FMO (FMO force field).
We also expect the participating researchers from industry to provide objective advice, from a practical viewpoint, on the calculation methods and results. FMODD will be the forum where we can exchange opinions concerning FMO and drug design.
Working groups:
Currently FMODD has eight Working Groups (WGs), three dealing with practical drug targets, two performing drug-design-related studies, and three developing computational methods for drug design. Each WG discusses and summarizes the results of the members, which will be brought together to the general meeting to share among all the members of FMODD. Each WG is headed by a coordinator or two from academia to promote discussion and cooperation among the WG members from industry, academia, and government.
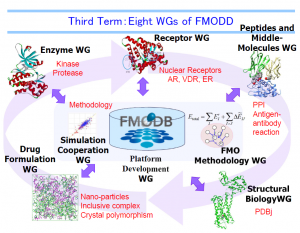
[Enzyme WG / Receptor WG / Peptides and Middle-Molecules WG / Structural Biology WG / Drug formulation WG / Simulation cooperation WG / FMO methodology WG / Platform Development WG]
Enzyme WG
-
Shigenori Tanaka
Kobe University -
Yoichiro YagiOkayama University of Science
Enzyme WG focuses on the FMO applications to proteases, kinases, and other enzyme-like proteins. As for kinases, we are investigating the binding energy of active compounds targeting p38 MAP kinase and JAK kinase, and evaluating the target specificity.
p38 MAP kinase is an example with available data on very diverse ligands. When the correlation between IC50 and FMO interaction energy was examined for 95 types of p38-ligand complex structures, no correlation was found at first, but the compounds were classified by scaffolds and in terms of the singular value decomposition (SVD) method for improving the correlations. It was then found that the “noise” that disturbs the correlation can be removed through these procedures and the value of the correlation coefficient is significantly improved. We published a paper [Sheng et al., 2018] summarizing how to make molecular modeling and classify the calculation results, and a paper [Maruyama et al., 2018] that improved the correlation with experimental values by the SVD for IFIE matrix.
Janus kinase (JAK) has four subtypes, JAK1, JAK2, JAK3 and Tyk2, and their target selectivity can be elucidated by the FMO method. We are also evaluating the effect of tyrosine phosphorylation on ligand binding, which is a characteristic of kinases. In addition, we are studying the prediction of IFIE values for ligand binding by machine learning.
As for proteases, we have performed the FMO calculation analysis for renin-inhibitor system, HIV-1 protease, HIV reverse transcriptase, and so on. We have then compared the calculated values with IC50 and other experimental results including the side effects.
FMO calculations for 20 renin-inhibitor complexes and 9 HIV-1 protease-inhibitor complexes have shown strong correlations between total IFIE (binding energy) and experimental activity (IC50 or Kd). As a result of clustering by VISCANA and detailed IFIE analysis of ligand and each amino acid residue of renin, it was suggested that the correlation between ligand structure and activity value can be analyzed by classification based on IFIE interaction pattern. The effect of crystallization water was also clarified.
Furthermore, we are working on the elucidation of the structure-activity relationship for the HIV-1 non-nucleic acid reverse transcriptase-inhibitor complex and the development of new derivatives that are effective for HIV-1 mutant strains.
Receptor WG
-
Noriyuki KuritaToyohashi University of Technology
We have conducted FMO calculations for the complexes of nuclear receptor proteins with various ligands. Our present target proteins are androgen receptor (AR), vitamin D receptor (VDR), retinoic acid receptor related orphan receptor gt (RORgt), and estrogen receptor (ER). We have also started classical MD simulations for the receptors to distinguish the different effects in the binding of an agonist and an inverse agonist on the structure of the receptors. The recent results for each target protein are shown below.
For AR, we first analyzed its binding properties with several existing ligands and confirmed that the FMO results can explain the trend of Ki values obtained by experiment [Kobayashi et al., 2017]. Furthermore, based on the FMO results, we proposed novel ligands with four types of substitution introduced into the functional group of the top-ranked existing ligand and analyzed their binding properties to AR to obtain a novel ligand with higher binding affinity to AR [Nakamura et al., 2021].
VDR has His residues near the ligand binding pocket, and their protonation states can affect the specific interactions between VDR and the ligand. Our FMO study [Terauchi et al., 2019] reveled that the chirality of the ligand and the His protonation state of VDR influence significantly the interactions between VDR and the ligand. This finding demonstrates the importance of correctly setting the protonation state of the His residue existing around the ligand when using a molecular simulation to analyze the interaction between the protein and various ligands.
RORgt has two types of effective ligands that act as an agonist or an inverse agonist. Although they are similar in structure, they have completely opposite actions on RORgt. To determine the cause of these actions, we conducted 300 ns MD simulations in water and analyzed the structural change of RORgt induced by the ligand binding. It was revealed that in the complex to which the inverse agonist was bound, the structure of Helix 12 (Figure A), which is involved in the transcriptional activity of RORgt, significantly fluctuated and transcriptional activity was suppressed. To evaluate the cause of this structural change, we performed FMO calculations on several characteristic structures; we found that rotation of the His479 side chain of RORgt triggered the structural change of Helix 12 (Figure B, [Suzuki et al., 2020] ). In future research, we intend to similarly analyze other ligands, and we aim to propose a ligand that can suppress the transcriptional activity of RORgt more effectively.
Figure (A) Specific binding between the Helix12 site of RORgt and a co-activator, (B) rotation of the His479 side chain and interaction changes between amino acid Tyr502 and ligand 3SX revealed by MD simulations.
ER, a female hormone receptor, has two subtypes (a and b), both of which have cocrystal structures with many ligands. ER-ligand binding has been extensively studied using FMO calculations to determine receptor binding affinity and subtype selectivity. To date, FMO calculations have been performed for cocrystal structures of ERa and 22 types of ligand, as well as ERb and 23 types of ligand. Based on the interaction pattern of IFIEs, we elucidated the ER residues important for the strong ligand binding and the selective binding to ERb [Seki et al., 2018].
Peptides and Middle-Molecules WG
-
Tatsuya Takagi, Osaka University
-
Norihito Kawashita, Kindai University
We have been dealing with various protein-protein interactions such as bromodomain, β-secretase, TCR-pMHC, FimH-mannose derivative, MDM2-p53, HIV gp120-anti gp120 antibody system, SARS-Cov-2 spike protein-antibodies. We are also investigating antigen-antibody reactions that lead to the evaluation of drug resistance, prediction of binding, and development of antibody drugs, as well as activity prediction by AI such as machine learning.
In the case of bromodomain system, we compared the interactions between peptide ligands and fragment compounds and analyzed the changes in the interactions during the design process of inhibitors using FMO method. As the binding affinity increases, the fragment compounds acquire interactions similar to those of the peptide ligands. We further analyzed CH/π interactions, charge changes, and the interactions between ligands and water molecules [Ozawa et al., 2017a, b].
In the case of TCR-pMHC system, we investigated the specific/non-specific recognition of T cell receptors (TCRs) and peptide-major histocompatibility complexes (pMHCs), which is important in immunological studies, focusing on CH/π interactions. PIEDA calculations were performed for each cocrystal-presented peptide, structures with the mutants (7, 8, 2, and 3 are replaced by 2, 3, 5, and 8, respectively) and the wild-type 105 (21 X 5) structure to clarify the interaction between each CDR and peptide [Tsuchiya et al., 2018].
Interaction calculations between the RBDs (antibody binding sites) of SARS-Cov-2 Spike protein and peptide antibody drugs showed that T415, K417, Y421, F456, A475, F486, N487, N501, and Y505 have important functions for the anti-viral activity. Among these, mutant strains of K417 and N501 have been reported to have an effect on infectivity and virulence, suggesting that changes (especially reduction) in the interaction are important factors in infectivity and virulence [Watanabe et al., 2021].
These FMO calculations for PPI suggest that mainly hydrogen bonds may be deeply involved in highly specific recognition and CH/π bonds in non-specific recognition.
Protein – protein interaction between SARS-Cov-2 Spike protein and antibody.
(left) PDBID:7CH5、(right) FMODBID: JM5M9
Structural Biology WG
-
Midori Kaminuma, Quantum-Structural Life Science Laboratories
The Structural Biology WG, which joined from the 3rd term, will proceed with research according to the following priorities with the aim of integrating the structural biology area and the computational science area, specializing in the following issues.
(1) FBDD using FMO (Explanation of X-ray analysis and NMR results by computational science)
For metalloproteases, which are often requested by the structural biologists, we will select LpxC as the first example. FMO adaptation for metalloproteases is also a common challenge for FMODD. It is an area for which there is no established method yet, and various cases should be considered for ligand protonation, which is a difficult task, but I would like to take on the challenge in close cooperation with the FMO Development WG. We would like to be able to explain the correlation between the inhibitory activity value (IC50) and IFIE and total energy calculated by FMO. As another example, we will proceed with FBDD, a compound that stabilizes PPI. It is a compound design that has the activity of becoming a compound (Stabilizer) that stabilizes the interaction with multiple proteins via the adapter protein 14-3-3. From the perspective of PROTACS, I think it is a target of interest.
(2) Down-sizing from Antibody / Peptide to small molecule (X-ray + Cryo-EM)
Reducing the molecular weight of peptides and antibodies is a supreme proposition, but it has not been successful yet . In setting the theme, as a result of recruiting within the WG, we decided to adopt IL2 low molecular weight. In addition, we will search for and proceed with high-quality targets.
(3) Membrane protein (X-ray, Cryo-EM)
Specific targets are still being selected, but we plan to focus on GPCRs.
(4) Collaboration with researchers in the field of structural biology.
Drug discovery research course by strengthening collaboration through meetings and seminars with researchers in the field of structural biology, including collaboration with the Japan Protein Data Bank (PDBj) and the Institute for Quantum Structural Biology of the CBI Research Organization established last year. We will proceed with initiatives that will be directly useful to.
Drug formulation WG
-
Kaori Fukuzawa, Osaka University
In the Drug Formulation WG, we are developing a method to utilize molecular interactions in formulation design by FMO calculations. We are developing simulation techniques for optimal formulation design, such as FMO-DPD calculations to elucidate phase separation behavior and nanoparticle formation processes, all-atom MD calculations to evaluate structure and physical properties, and FMO calculations to quantitatively evaluate molecular interactions. The activities of the Formulation WG are conducted in collaboration with experimental studies, and repeated cross-validation leads to the development of practical technologies. Currently, four themes are being promoted.
(1) Evaluation of crystal stability and conformer screening
(2) Molecular states and phase separation behavior in solid dispersions
(3) Design of lipid nanoparticles
(4) Molecular behavior of inclusion complexes
Phase separation behavior of solid dispersions (left), structure of lipid nanoparticles (center), and intermolecular interactions inside crystals (right).
Simulation cooperation WG
-
Koichiro Kato, Kyushu University
We have been developing methods to cooperate with various simulations and FMO. In collaboration with the KBDD consortium, we have performed FMO calculations for a large number of MD sampling structures of protein-ligand hydration complexes [Araki et al., 2016], and developed a highly accurate activity prediction method based on these calculations (see Fukuzawa et al. “FMO Drug Design Consortium” in [Mochizuki et al., 2021]). Currently, we are also working on the use of machine learning. In collaboration with PJ14 of the Life Intelligence Consortium (LINC), we are developing high-precision molecular force fields and interaction prediction models by combining FMO methods and machine learning. By using the FMO method, it is not only possible to create high-precision training data for giant heterogeneous systems such as protein-ligand complexes, but also to obtain IFIE and PIEDA. We are leading the world in simulation cooperation that takes advantage of the features of FMO. We have also succeeded in developing a machine learning model that can accurately predict RESP atomic charges obtained by the FMO method for the purpose of upgrading existing molecular force fields [Kato et al., 2020].
FMO methodology WG
-
Tatsuya Nakano, National Institute of Health Sciences
We have been developing the FMO-dedicated programs, ABINIT-MP and BioStation Viewer, while concurrently improving FMO methodology for drug discovery, such as inclusion of solvent effects and efficient structure optimization via the FMO method.
We have been developing the optimization technology for the evaluation of protein-ligand binding ability as an important issue for the practical application of FMO drug discovery, because the quantum mechanical structural relaxation around the ligands enhances the activity correlation. In collaboration with the AMED-BINDS project, we have attempted to speed up the process by introducing the flow domain method and redundant coordinate transformation. For the practical application of this substructure optimization technology, we are working on the validation of various protein-ligand complex systems. We are also working on the development of faster and more sophisticated FMO calculations coupled with a continuous dielectric model describing the in vivo environment, as well as structural optimization techniques that include environmental fields. In order to improve convenience, we will also expand the number of supported residue species including nucleic acids in the automatic fragmentation function.
We are developing BioStation Viewer, which enables input file creation and result visualization for ABINIT-MP specific input/output formats such as fragmentation and fragment interaction analysis required for FMO calculations. BioStation Viewer is available free of charge on this website.
Molecular operating environment (MOE) is a highly scalable and sophisticated molecular modeling software based on the original language, scientific vector language (SVL). We are developing FMOe that can complete the FMO calculation workflow (input file creation and result visualization) on MOE. The input file creation interface supports automatic fragmentation and manual fragmentation of proteins, and the visualization interface allows interactive visualization of IFIE/PIEDA obtained from FMO calculation results.
Interfaces for generation of input file (left) and visualization of output file (right).
Platform Development WG
-
Teruki Honma, RIKEN
We will develop a platform for FMO drug discovery, such as developing FMO database, collecting active data, developing an FMO automatic calculation protocol that automatically processes complicated calculations, and MD sampling data flow, and in collaboration with AI technology. We are aiming to develop methods to design new drug candidates instead of retrofitting.
The detailed items currently under development are as follows. The names in parentheses are the main development organizations.
・ FMO automatic calculation protocol (RIKEN)
・ FMO database (FMODB, RIKEN, Hoshiyaku University)
・ Activity prediction by FMO-AI (RIKEN, Sumitomo Chemical)
・ FMO grid and its applications (RIKEN, Konan University)
・ FMO force field (RIKEN, Kyushu University, LINC)
FMO automatic calculation protocol – A protocol that semi-automatically performs complementation of protein structures including ligands, fixing protonation/deprotonation/tautomerization states, structure optimization, creation of inputs for FMO calculations, calculation execution, and extraction of interaction data from calculation results [Watanabe et al., 2019]. Using ERα and p38 MAP kinase, we have confirmed that it is possible to perform calculations with accuracy comparable to the manual calculations of experienced FMO researchers, and the majority of the data in the FMO database exceeding 10,000 is calculated by this protocol. The protocol uses the functions of commercial software, but is currently developing a replacement version with free software.
FMO database (FMODB) – FMODB is the world’s first database containing quantum chemistry calculation results of proteins, and was released to the public in February 2019, and in March 2020, the data of COVID-19-related proteins were released and a press release was made [Takaya et al., 2021]. As of April 2021, it contains FMO data for 13,050 protein structures. Currently, high-resolution protein structure calculations are systematically performed in cooperation with structure WG and PDBj.
FMODB Home Page – https://drugdesign.riken.jp/FMODB/
Affinity prediction by FMO-AI, FMO grid and its applications – FMO-AI is a generally called method for constructing a highly accurate affinity prediction models by performing machine learning using the energy values of interactions obtained from FMO calculations of complex structures of various ligands and proteins. In addition to PIEDA, we are also developing descriptor sets that reflect the 3D alignments of interaction energies used for learning [Kato et al. 2020]. One of them is the FMO grid, which places probes such as methane at grid points in the pocket and calculates the interaction energy in advance.
FMO force field – In collaboration with the simulation WG and LINC, we are developing a new force field that can more precisely reproduce quantum mechanical interactions than the conventional AMBER-based molecular force field. We have already developed AI prediction models that reproduce the FMO charge and published a paper. We have performed tens of thousands of FMO calculations for the AI prediction model, and part of that data is also published to FMODB.
Fragment Molecular Orbital (FMO) method
It is difficult for conventional ab initio quantum chemical methods to handle biological macromolecules such as proteins and nucleic acids because of the enormous amount of calculation needed. To overcome this difficulty, in the FMO method, macromolecules are divided into small fragments, the electronic states of fragments and fragment pairs are solved in the presence of environmental electrostatic potential from the surroundings, and the entire electronic state is constructed by combining them.
Simultaneously, the interaction energy between fragments (Inter-Fragment Interaction Energy; IFIE or Pair Interaction Energy; PIE) and its energy components (Pair Interaction Energy Decomposition Analysis; PIEDA) can be obtained as by-products of the FMO calculation [1] [2] [3].
Both IFIE and PIEDA are extensively used in in silico drug design to evaluate the binding (free) energy and molecular interaction between ligands and target proteins. The effects of thermal fluctuation of the molecular structure in solvent can be incorporated by several appropriate methods.
[1] K. Kitaura et al. (1999) Chem. Phys. Lett. 313, 701-709 https://doi.org/10.1016/S0009-2614(99)00937-9
[2] D. G. Fedorov et al. (2012) Phys. Chem. Chem. Phys. 14, 7562-7577. https://doi.org/10.1039/C2CP23784A
[3] S. Tanaka et al. (2014) Phys. Chem. Chem. Phys. 16, 10310-10344. https://doi.org/10.1039/C4CP00316K
Promotion of HPCI
High Performance Computing Infrastructure (HPCI) is a system that connects the supercomputer “Fugaku” with the computing resources of universities and research institutes nationwide via a high-speed network. FMODD has been conducting a research project named “Construction of an FMO drug design platform utilizing HPCI” since FY2015, using K-computer (RIKEN), Tsubame 3.0 (Tokyo Tech.), and FX100 (Nagoya University).
FMODD utilizes and promotes HPCI.
Kaori Fukuzawa, FMODD Chair, discussing FMODD and HPCI in the symposium “Talking about HPCI (in Japanese).” https://fugaku100kei.jp/hpci/